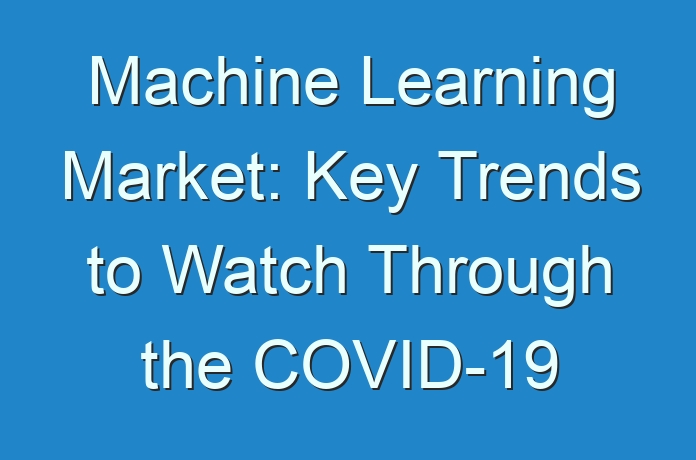
ML Accelerating COVID-19 Research and Identifies Patterns in Data
Machine learning (ML) and artificial intelligence (AI) technologies are being deployed in areas of research to fight the COVID-19 pandemic. This research is being extended toward healthcare and agriculture, as currently these are one of the most essential industries during unprecedented times. ML is being highly publicized since this technology enables computers to mimic human intelligence and ingest large volumes of data in order to identify patterns and insights. Thus, companies in the machine learning market are capitalizing on this opportunity to scale customer communications and speed up COVID-19 research for treatment.
The ML technology is bringing quarantine measures and social distancing in place for employees with the help of remote communication, telemedicine, and food security. The proliferation of ML in virtually all end markets is creating value-grab opportunities for companies in the machine learning market.
Request a sample to get extensive insights into the Machine Learning Market
Facial Recognition Systems Acquire Prominence in Banking Sector to Prioritize Assistance
The banking sector is able to convert data samples into new interfaces with the help of machine learning. As such, the machine learning market is predicted to cross the value of US$ 600 Bn by the end of 2030. Nowadays, ML has become a prerequisite feature for any intelligent system in the banking sector. ML is facilitating data-driven predictions that help to generate new business opportunities for individual institutions. ML is gaining popularity as the branch of AI that can be used to improve products and customer services in the banking sector.
Automatic reply predictions for email messages and virtual assistants have become customary in the banking industry. Companies in the machine learning market are innovating in facial recognition systems to capitalize on revenue opportunities in the banking sector. These systems help to prioritize individual data before a consumer approaches a banker in an institution for assistance.
Proper Training for Algorithms Improves Computational Feasibility on Large Datasets
The machine learning market is expected to advance at an explosive CAGR of ~27% during the forecast period. However, the implementation of ML models is emerging as a challenge for companies. For instance, computations with Big Data can lead to issues in processing performance of ML models, thus making even trivial operations expensive. Companies in the machine learning market should provide proper training for algorithms in order to improve computational feasibility on very large datasets.
On the other hand, many business owners have stated that time-consuming deployment of ML is predicted to impede market growth. Hence, software companies are educating business owners to deploy ML models on a small scale to check its feasibility. As such, companies are tapping into incremental opportunities in cybersecurity to help business owners identify fraud and prevent phishing attacks.
ML Helps to Maintain Equilibrium between Energy Supply and Demand
Renewable energy forecasting and predictions regarding power output are some of the useful applications of machine learning. Forecasting has become necessary in the energy and utilities industry, since key renewable energy sources i.e. wind and solar are variable in nature and are dependent on external factors. Germany is known as the poster child for adoption of renewable energy. However, the intensity of solar radiation and wind speed is never uniform. Hence, companies in the machine learning market are proving their software and services to energy and utilities companies to maintain an equilibrium across energy supply and demand.
The machine learning market is dominated by established players such as IBM. However, emerging market players such as the U.K.-based AI company DeepMind, was recently acquired by Google and is posing as a stiff competition to established market players.
Stuck in a neck-to-neck competition with other brands? Request a custom report on Machine Learning Market
Game-changing Insights on Customer Behavior Benefit Retailers and Telecom Companies
ML models are helping retailers to better understand and analyze customer behavior data, which is collected from various trends, demographics, and preferences. Personalized experiences are becoming increasingly important in the retail industry. With the help of old data, ML models help to predict, which product is worthy of recommendation for customers. The burgeoning growth of the eCommerce sector is acting as a key driver for the expansion of the machine learning market. Retailers are able to analyze digital behavior patterns of customers in order to improve their purchase journey.
Real-time insights powered by ML and AI are grabbing the attention of companies in the IT & telecom sector. Moreover, IoT (Internet of Things) and 5G technologies are fueling the growth of the machine learning market. ML algorithms are being used by telecom companies to track data streaming from a plethora of network interfaces.
Automakers and Digital Operators Adopt ML Tools to Enable Productive Consumer Involvement
Apart from retail and banking, companies in the machine learning market are broadening their revenue streams in automotive and content moderation applications. The trend of autonomous vehicles is gaining strong business grounds worldwide. It has been found that self-driving cars will help to drastically reduce traffic-related issues. Software engineers are relying on ML algorithms to design autonomous features in self-driving cars in order to make the vehicles more consumer-centric.
High accessibility of the Internet and modern technology have led to the outspread of fake news and disinformation. In such situations, content moderation comes into play where ML platforms are enhancing interactions between humans and AI to enable the content moderation task. Social networking platforms and news outlets are adopting ML models to increase human involvement toward productive work. ML tools help to address and analyze the context of every single frame of video content in order to drive content moderation.