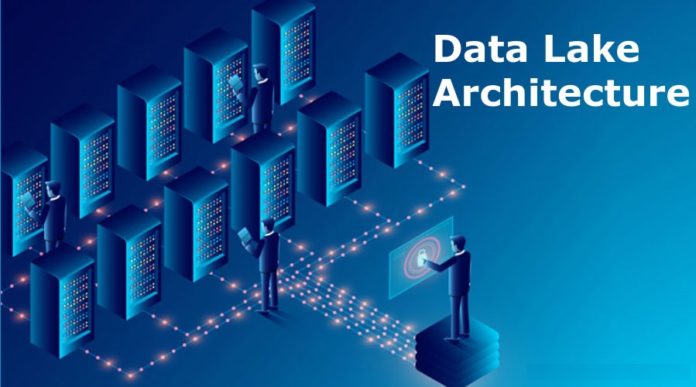
The start of the new millennium of data science has added new dimensions to the concepts of big data, fast data, and data lakes. In the present times, data acts as a prime asset for rapid decision-making processes. A study by IDC titled, “The digital Universe of opportunities” concluded that the level at which unstructured data is growing is sufficient to cross the mark of 50 ZB by 2050. That said, the number of applications that would depend on big data and fast data would increase by a large amount.
In this article, we take a look at the changing dynamics of big data along with the data lake architecture. We conclude with fast data processing capabilities for the future.
The changing dynamics of big data analytics and three major principles
Big data can be related to such datasets which can’t be analyzed with the capabilities of traditional data storage facilities. This means that big data cannot be processed using the available tools in a reasonable amount of time. Let us briefly define three major types of big data processing. The first is batch processing which is also called real-time processing. In this type of processing, data that is stored in temporary memory is processed at the first instance. After this, the data processing and data conversion processes are undertaken. These processes are highly influenced by the type of operational problems in question. The second type of processing is called stream processing. In this processing, data is collected without the need to store it in a particular device. However, the results obtained by processing operations need to be stored for further processing. Stream processing is needed when the rate of response time needs to be as low as possible. The last type of processing is called hybrid processing which works on three major architectural principles. The first principle is that of precision. This means that human and other types of errors are avoided by this principle. The second principle works on the immutability of data. This means that raw data is to be stored in its original format irrespective of the number of modifications that are performed from time to time. This original format cannot be altered at any point in time. The last principle works on a low latency rate. This means that the time elapsed between the processing of data and yielding of results is kept as low as possible.
The concept of a data lake
Data Lake is a concept that was introduced by James Dixon in the year 2010. The commercial viability of data lake spans from the fact that it supports Hadoop. A data lake has undergone great development in the present times because it supports a message scalable repository for the storage of gigantic amounts of data in its raw format. A data lake is also able to handle a voluminous amount of unstructured data from which deeper insights can be derived. The components of the data lake include a semantic database which can be used to create relationships between various data sets by manipulation techniques. The interrelationship of one data set with a completely different data set can also be established in terms of similarity index. The best thing about data lake is that it can amalgamate structured query language and online analytical processing capabilities.
Deep diving into a data lake
In times where the internet of things is the new normal, a data lake can be used to store logs and sensor data. With the help of data lake, we can perform analytics by utilizing data workflows and subject-specific case studies. It increases our processing capabilities by avoiding duplication and reducing latency time. The ease of access from the remote test platforms is another advantage of this concept. Access to various modules can also be provided with the help of multiple parties to manage and work on a project at the same time. The data cardinality is an added advantage that speaks about the relationship between two hitherto unrelated data sets.
Conclusion: Fast data for future
Various streams of data analytics that flow from the internet of things in the present time have to be subjected to processing in the future. The concept of fast data focuses on the analysis of relatively small amounts of data in real-time. This concept is particularly helpful for solving a complex problem with high precision. One of the prime characteristics of fast data is the speed at which streams of data are analyzed. The goal of fast data is to work on the minute quantum of data surgically. When data is sensitive, fast data finds tremendous applications in this case. Fast data processing is particularly suitable when rapid action needs to be taken on a sample of data that is being constantly monitored. In one word, fast data would take the concept of big data analytics to the next level in near future.