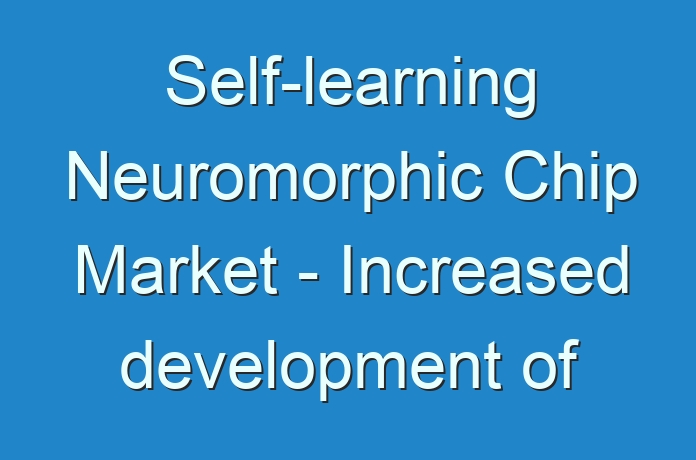
Self-learning Neuromorphic Chip Market: Introduction
- Neuromorphic chips come with artificial neurons and artificial synapses that mimic the activity spike that occurs in the human brain. The chip has the ability to learn continuously due to its synaptic plasticity. This results in smarter, far more energy efficient computing systems.
- Self-learning neuromorphic chips perform on chip processing asynchronously. It uses event driven processing models to address complex computing problems. Further, by combining improved on board learning, reduced latency, and improved energy efficiency, the self-learning neuromorphic chip can push the image recognition and speech processing to new levels of speed and accuracy.
- The field of self-learning neuromorphic chips is advancing rapidly and it is expected that the chip will have a positive impact on edge devices and IoT systems. Research and developments are being done by companies, for instance, Intel Labs has developed Loihi which is a research test chip. The chip uses spiking neural network architecture.
Want to know the obstructions to your company’s growth in future? Request a brochure @ https://www.transparencymarketresearch.com/sample/sample.php?flag=S&rep_id=71493
Growing adoption of self-learning neuromorphic chips in automotive industry
- The automotive industry is among the fastest growing industries in the world. It is showing rapid adoption of neuromorphic chips in automated cars. Premium car manufacturers are investing in automotive technology to achieve level five automation. This in turn is generating huge demand for self-learning neuromorphic chips.
- In addition, neuromorphic chip developers are focusing on advanced driver assisted system (ADAS) and autonomous vehicle (AV) applications to increase their market reach. These factors are fueling the growth of the self-learning neuromorphic chip market.
Increased development of self-learning neuromorphic chips by manufacturers
- Neuromorphic chips are being developed by major as well as emerging players across the world. For instance, IBM has developed a neuromorphic chip named TrueNorth which has 4,096 cores, each with 256 neurons that in turn, contain 256 synapses each. Moreover, AI startups across the world are engaged in development of neuromorphic chips and technology for a wide array of purposes.
- Companies are also developing software to optimize the performance of the chip. For instance, Applied Brain Research has developed software to program neuromorphic chips. These developments are propelling the market growth.
Maximum Growth to be observed in the Asia Pacific Market
- In terms of region, the global self-learning neuromorphic chip market can be divided into North America, Europe, Asia Pacific, South America, and Middle East & Africa.
- The self-learning neuromorphic chip market in Asia Pacific is anticipated to expand at the maximum CAGR during the forecast period.
- This growth is attributed to rising adoption of self-learning neuromorphic chips in automotive, healthcare, and other industries, benefits of self-learning neuromorphic chips, and technological advancements. These factors are expected to drive the demand for self-learning neuromorphic chip products.
- The self-learning neuromorphic chip market in North America and Europe is expected to show high growth rate due to increasing competition among players. The market in Middle East & Africa is likely to show slow growth due to lack of investments in R&D.
Purchase Premium Research Report @ https://www.transparencymarketresearch.com/checkout.php?rep_id=71493<ype=S
Key Players in the Global Market
The global self-learning neuromorphic chip market is highly concentrated as limited number of manufacturers are present. Prominent players operating in the global market are focusing on product launch and technological developments to meet the growing demand.
Key players operating in the global Self-learning neuromorphic chip market include:
- Intel Corporation
- IBM
- Qualcomm
- HRL Laboratories
- Hewlett-Packard
Read Our Trending Press Release Below: https://www.prnewswire.co.uk/news-releases/with-growing-focus-of-enterprises-on-technological-advancements-magnetic-flow-meter-market-likely-to-grow-at-6-cagr-tmr-876969375.html